Early intervention is one of the most effective ways to prevent academic failure and school dropout. But figuring out who needs help can be challenging, and even when you have the data to identify which students are at risk, what do you do then? This is where an Early Warning System (EWS) comes in! More than just a data set, an effective EWS uses attendance, behavior, and course performance indicators to identify students at risk of school failure or of not graduating on time and helps educators intervene to change the trajectory.
Use this guide to help your school improve educational outcomes for all students, especially those in danger of not graduating, using an Early Warning System.
This EWS Guide Uncovers:
-
Comprehensive steps to build and implement an effective Early Warning System tailored to your school’s needs.
-
Expert tips on interpreting data to accurately identify at-risk students.
-
Proven strategies to engage and support students, improving retention and outcomes.
-
Dive Deeper! Download Full EWS Guide
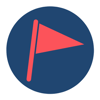
Early Warning Systems
Despite growing investments in education, student dropout rates continue to be a global issue. In the United States, 1 in 5 students do not complete high school. The risk of not graduating is even higher for students from marginalized demographic groups, where high 9th-grade retention rates increase the chance of dropping out before graduation. In some schools, labeled “dropout factories,” the student population decreases more than 40% from 9th to 12th grade.
Early warning systems (EWSs) aim to identify students at risk of not graduating on time, enabling schools to implement targeted interventions that reduce dropout rates and support positive student achievement. A well-implemented EWS uses a systems-level approach to integrate key data points to understand areas where support and improvements are needed.
There is no one-size-fits-all approach to developing an effective EWS; districts may use different indicators to measure, assess, and understand the prevalence of risk factors for not graduating among their population of students. Nevertheless, some key recommendations and guidelines can be used in developing an EWS to ensure the adequate allocation and implementation of resources to prevent student dropout.
Research on EWS
Research on EWSs in schools demonstrates their effectiveness in identifying students at risk of academic failure or dropping out. These systems have shown promise across several studies:
-
One study found that middle and high schools implementing EWSs had significantly more students without early warning indicators at the end of sixth and ninth grade; the most substantial impacts were observed in sixth grade, where chronic absenteeism was significantly reduced5.
-
Faria and colleagues provided further evidence in a study involving 73 high schools, revealing that EWSs had a significant positive effect on reducing chronic absenteeism and course failures.
-
Similarly, Davis and colleagues found that high schools with strong implementation of the core EWS components in ninth grade experienced greater improvements in student attendance and course performance.
These studies underscore the promise of EWS in helping schools identify and support at-risk students early, leading to better academic and behavioral outcomes. Despite positive results, challenges remain in fully integrating EWS into school systems, including ensuring equity in support and addressing resource constraints for effective intervention implementation.
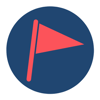
Early Warning Indicators (EWIs)
Early Warning Indicators are the central component of Early Warning Systems. Early Warning Indicators (EWIs) function as red flags, helping to identify students at risk of falling behind or dropping out altogether.
Many factors can be used as indicators by schools and districts, but they must be linked to student outcomes. EWIs are used to identify students early on and provide targeted interventions. and support to help them stay on track toward their educational goals.
Important note: It is more effective to use EWIs than to rely on tracking academic risks based on student demographics. Certain demographic characteristics, such as race, ethnicity, and socioeconomic status, can be correlated to educational outcomes; however, they are not strong predictors on their own. Relying on these factors can also lead to biases in decision-making.
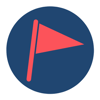
Early Warning Systems and MTSS
In a Multi-Tiered System of Supports (MTSS), academic and behavioral data are leveraged to guide decision-making and help educators meet the needs of all students. Thus, EWSs can be used within an MTSS to provide educators with the information they need to identify students needing additional support or intervention. Nevertheless, many educators struggle to integrate their EWS within their MTSS practices, and as a result, these systems remain separate.
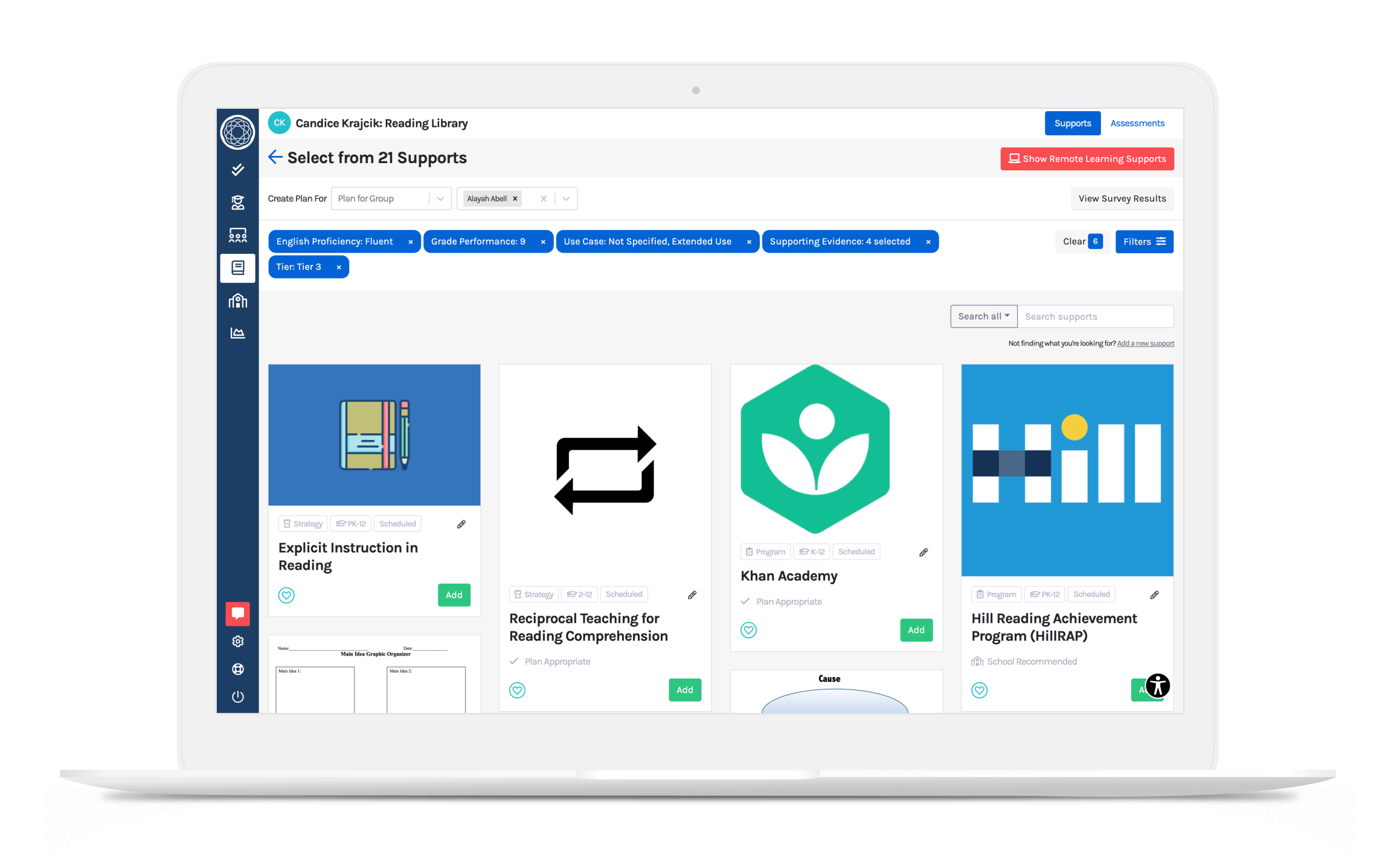
Library of Hundreds of Curated MTSS/RTI Evidence-Based Interventions
The Branching Minds Library of Supports includes hundreds of evidence-based intervention programs, so if your school or district has purchased those resources, they can be added to a student’s intervention plan on Branching Minds, as well as nearly a thousand free evidence-based strategies, activities, and resources that can be added to a student’s intervention plan.
EWS and Branching Minds
Branching Minds’ MTSS Solution helps educators proactively identify and support at-risk students by pulling together crucial data points for student success and making them visible across a student’s support network. Our Early Risk Report functionality gives teachers insights into student risk levels across attendance, behavior, and academic performance—data points that research consistently shows to be predictive indicators of student dropout. This approach supports the success of the whole student, allowing individual teachers and school systems to see where their student body is at risk, understand the barriers, and proactively deploy resources and interventions for the students in greatest need of support.
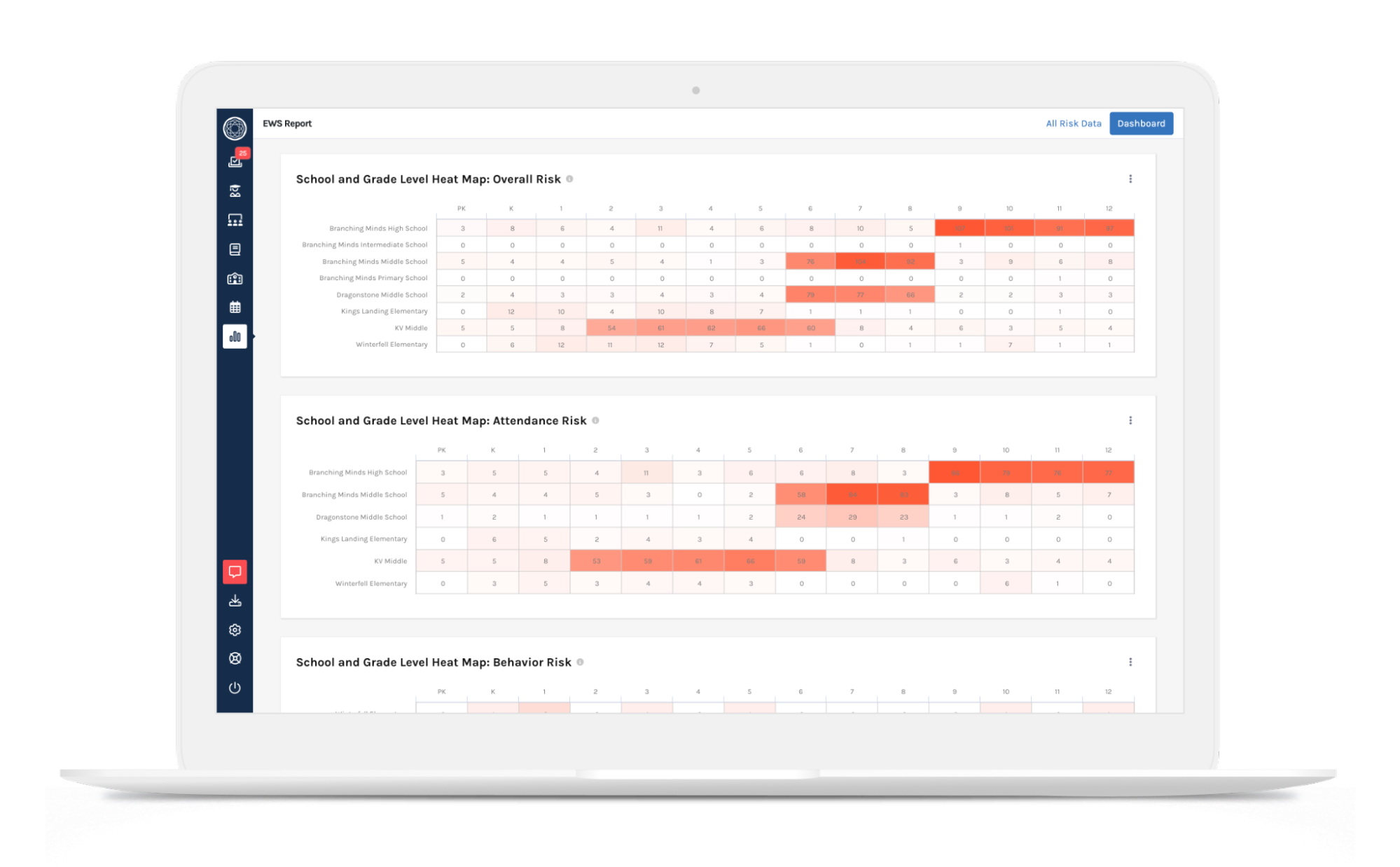
For school and district leadership: The Graduation Risk Report provides an aggregated view of risk to inform system-level changes that can better support teachers and students.
Learn more about how your school can use Early Warning Systems to predict student dropout and empower your educators to provide targeted support to students at risk.
EWS |
Podcast: Podcast Episode with EWI Expert Sarah Frazelle
|
|
Webinar: Early Warning Systems and MTSS: How to Flag and Prevent Student Failure |
||
Video: What is an EWS? |
||
Infographic: Introduction to EWI |
||
Blog: What Are MTSS Assessments in High School, and Why Are They Important? |
||
About the Author
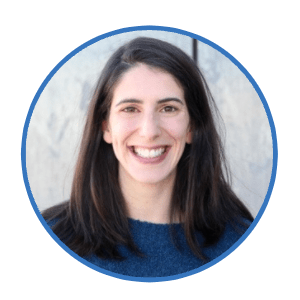
Essie Sutton, PhD
Dr. Sutton is an Applied Developmental Psychologist and the Director of Learning Science at Branching Minds. Her work brings together the fields of child development and education psychology to improve learning and development for all students. Dr. Sutton is responsible for studying the impacts of the Branching Minds platform on students’ academic, behavioral, and social-emotional outcomes. She also manages and curates the platform’s library of evidence-based supports and studies how different strategies, programs, and tools impact students’ educational outcomes.
Dr. Sutton received her Ph.D. in Applied Developmental Psychology from Fordham University, where her research focused on evaluating academic and social-emotional learning programs in elementary classrooms. Her work has also examined how classroom contexts and the quality of teacher-student interactions influence students’ learning and social development. She has also developed and studied assessments used to measure indicators of children’s well-being and teachers’ classroom practices. Dr. Sutton’s research has been published in Child Indicators Research and the Journal of Applied Developmental Science. She has also presented her work at national conferences, including the American Education Research Association and the Society for Research on Child Development. Dr. Sutton also has an M.A. in Human Development, Learning, and Culture from the University of British Columbia and a B.A. from McGill University.